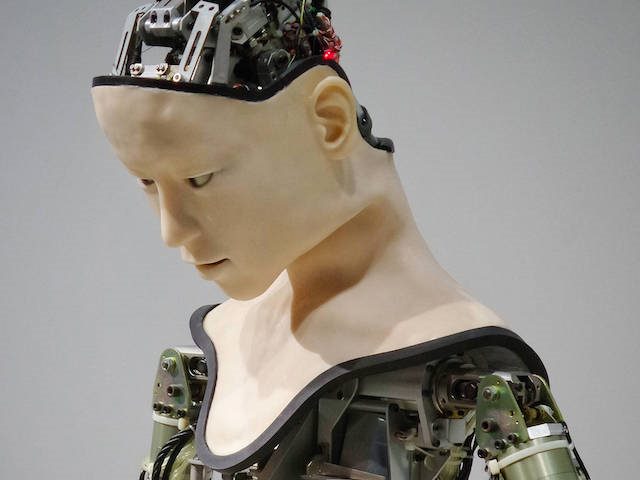
Originally published on CMSwire.
As the business world grapples with the potential of AI and machine learning, new ethical challenges arise on a regular basis related to its use.
One area where tensions are being played out is in talent management: between relying on human expertise or in deferring decisions to machines so as to better understand employee needs, skills and career potential.
Companies like IBM, with a workforce of 350,000, are at the forefront of employing new technologies and techniques including machine learning and AI to help recruit and retain the right kinds of workers.
AI and HR, Perfect Together?
When IBM CEO Ginni Rometty was recently interviewed at a work and talent summit, she relayed the full extent to which the company is committed to using AI to realize these goals.
IBM is no stranger to the AI space, having built up considerable expertise (and market share) with its powerful Watson AI service.
As Rometty outlined, the company has built new algorithms and services to recruit candidates, to spot which employees should be trained and equipped with new skills, and for some unlucky souls, to identify those who no longer fit what the business needs. She even claimed they can now predict with a “95% accuracy” which employees are likely to leave their jobs.
What drove all this? Beyond responding to new business models and emerging technologies (including machine learning and AI), internally the company needed to shake up how its teams operated. Rometty said they realized they could no longer rely on manager’s abilities to assess their team’s performance through use of formal skill assessments, whose results were deemed too subjective and not entirely accurate.
Instead, she said, “We can infer and be more accurate from data.”
This meant being systematic in matching employees against what the business needs from them: on what they currently can do, on their capabilities, and where opportunities might lie ahead.
As it turned out, this approach had other benefits too. The company chose to stop relying on what can be cumbersome, bureaucratic annual performance reviews. Instead, they set about assessing employees based on their skills growth.
Every employee also gets access to IBM’s MYCA (My Career Advisor) powered by Watson, which acts like their personal career advisor, “to help employees identify where they need to increase their skills” and elsewhere serves up appropriate job openings.
Why Businesses Aren’t Handing HR Over to AI
Yet, amidst the techno-fueled hubris, the author of the Rometty article, Eric Rosenbaum, concluded with a cautionary coda: “IBM’s bet is that the future of work is one in which a machine understands the individual better than the HR individual can alone.”
A sobering thought, but perhaps unrealistic too? As it turns out, three major reasons are stopping other businesses from following suit — or for now, at least.
First, unlike IBM, many are ill equipped. As KPMG reports, only 36% of the 1200 HR executives interviewed have started to introduce AI and feel they are suitably equipped with the necessary skills and resources to make use of it. It’s early days, and the technology is largely an unknown.
Second, some think the technology threatens the central role HR plays in business. As Adina Sterling, an assistant professor of organizational behavior at Stanford Graduate School of Business explained in Stanford Business, “Hiring demands a global view of the company and its direction within a shifting market. Computers do not possess such a view.”
For example, she questions whether AI has the smarts to spot outlier candidates — those with “unusual talent” — who may not fit a standard model, but who can bring new skills and expertise, or equally deserve to be nurtured.
But Sterling’s central point is that HR needs to “have the sense that they’re held accountable for what algorithms are doing” and not the other way round. She cautions HR against being fooled by the technology and ending up relinquishing their strategic focus.
The third issue with AI — and perhaps the biggest one — is that few fully understand how AI actually works. It’s AI’s Achilles heel. Algorithms are complex beasts and operate in black boxes, unfathomable to most people. Put another way, they are akin to Polanyi’s paradox: how they function is “beyond our explicit understanding.”
AI’s Lack of Accountability
However, this needn’t be a problem if you’re happy with the calculations, models and predictions it comes up with. Why question something that seems correct?
Issues can arise, if for example, an IBM employee decides they don’t like how they’ve been categorized; dislikes a suggested training pathway; or asks why they appear to be on the way out.
Here things can get tricky. Because if no one — including managers — has an inkling of how such decisions have been made, how can you expect to trust it? And more worryingly, how can you challenge or refute it if you have no idea how it works?
The problem with AI in this sense is its unaccountability. Being unable to explain it puts it beyond reason.
Algorithms are very complicated things: they rely on datasets, need to be trained and supplied with rules to work. Whether they use deep learning, Bayesian probability, or some other kind of statistical calculation, they can be hard to fathom.
What happens between when the program is turned on, to its end point, can be a complete mystery — even to the programmers who have created it.
To their credit, some have begun to recognize the seriousness of this problem. IBM in 2018 sought to demystify and explain how its own algorithms work, albeit with a slightly clumsily titled “Trust and Transparency capabilities for AI” initiative.
Tellingly, DARPA (the hugely influential and secretive US defense research agency) has launched its own AI PR project, with a new scarily named research arm called Explainable Artificial Intelligence (XAI).
Gaming the System
These initiatives go some way to humanizing AI. However, for some, like researcher Sandra Wachter, they don’t go far enough and different ideas are needed to unravel more of their complexity.
A professor at Oxford Internet Institute, Wachter offers a more novel approach, which relies on gaming the algorithms to encourage users to test them out, play with them and understand why they model outcomes in the way they do.
Wachter believes in keeping AI’s black boxes intact, but argues we need to come up with what she calls “counterfactual explanations.”
An example could be an employee who didn’t receive a promotion as a result of an algorithm-based model. Wachter’s idea is to let the employee find out what would have been the “smallest possible change that would have led to the model predicting a different outcome.”
For example, which different skills or competencies would have made all the difference to the employee’s scores? It’s a canny solution: it keeps the algorithms’ IP secret, whilst letting users model different scenarios. This approach can also help test for fairness too.
As she says, it would be a “major step forward in terms of transparency and accountability.” She also goes on to warn about needing more transparency over which data points are being used to profile individuals and “train decision-making algorithms.”
This is another huge area to consider. It’s one thing to know how an algorithm works, it’s entirely another to know what kinds of data is being collected from you and why — often, without your prior knowledge.
Gains and Losses, But at What Cost?
True, IBM has demonstrated success is possible in using AI, claiming it has saved them nearly $300 million in retention costs. Yet that process also meant (perhaps ironically to some) they reduced their HR headcount by some 30%.
When asked, tellingly Rometty refused to explain “the secret sauce” of how the algorithm works. Some might say that is very convenient, especially during what must have been a difficult cost-cutting exercise — in effect putting the rationale and accountability of deciding who loses their job beyond the reach of their human managers.
But isn’t that also known as passing-the-buck?